What is credit scoring?
Credit scoring is a tool for segregating client credit exposures based on expected default behavior. It uses a number of techniques to grade clients based on their credit worthiness. From a portfolio and risk management point of view it also identifies relationships that the bank should invest in retaining as well as accounts that need to be closely watched and monitored.
The challenge with credit scoring?
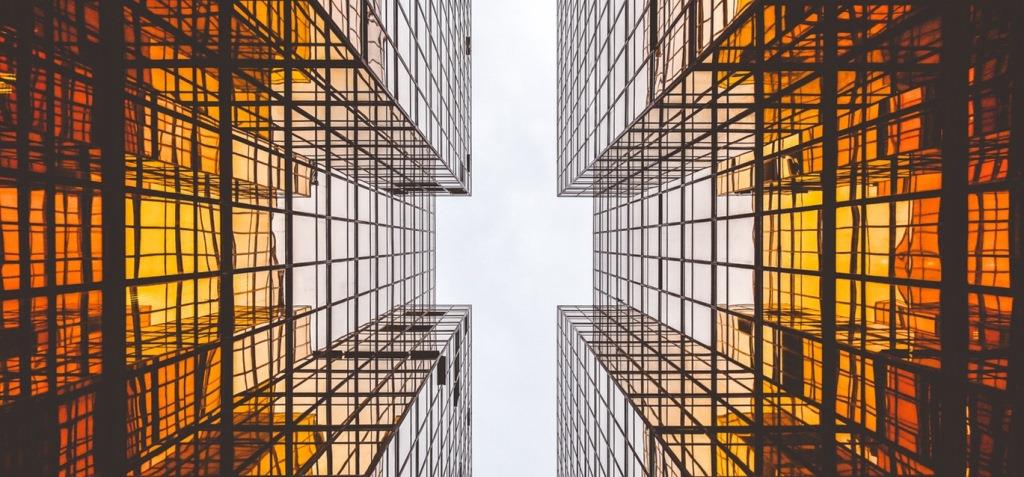
For corporate customers credit scoring models rely heavily on client financial data. While a number of non-financial parameters are also considered, models tend to give more weightage to financial data which in many emerging market is contestable. In such markets clients maintain multiple books to reduce the burden of taxes or avoid regulatory oversight. Published and audited financial statements are primarily used for public consumption and as such may not truly reflect the correct situation on the ground for a client.
Financial data is also reviewed on a yearly basis so erratic customer behavior during the year may not lead to a change in the client’s score and how the relationship is managed. While banks have multiple tools for managing the relationship and taking actions when payments are delayed these generally get triggered once certain payment thresholds are crossed. Client files are reviewed when their payments cross acceptable thresholds. Making such actions reactive rather than preemptive in nature.
Typically credit scoring engines use an application score approach. The lending application is scored based on historical client data. It includes a combination of subjective and objective customer attributes such as segment, sector, industry, and quality of auditors as well as multiple financial ratios and their relative ranks across industry and segment averages. An alternate approach made common by consumer scoring model is behavioral models that use a combination of historical payment related behavior to determine consumer credit score and rank across the portfolio. It is common to use a number of derived payment statistics such as number of late payments in a year, number of consecutive late payments, average days past due series and comparison of these derived statistics with bank portfolio benchmarks.
Corporate, commercial and SME portfolios use payment behavior specifically days past due data for collection management. In addition raw payment behavior information is available through the accounting system and can be used to calculate derived statistics similar to consumer portfolios.
Credit scoring. The alternate approach.
While the use of financial ratios in allocating credit scores to corporate customers is an accepted practice, payment behavior usage for non-retail banking customer credit scoring is limited. It is interesting that this is the case despite the fact that Robert Altman who introduced the usage of Altman Z-scores for corporate customers also used repayment data for bonds portfolios to predict the rate of default.
Using historical client financial information data for scoring purposes has a number of issues. Unless the bank in question has invested in a loan origination or credit scoring application, historical financial data for customers may only be available in paper files and needs to be captured, encoded and stored in proper templates before it can be used for credit scoring. The original Altman Z-Score applied to a given data set. Model parameters and coefficients for Z-Score need to be identified and estimated for each dataset. A bigger challenge is the ability of financial data to accurately predict and forecast the true state of finances at the client. Even without the distortions in emerging market data sets mentioned above, financial data is a lagging indicator of current or future financial performance. It is a point in time snapshot that for credit purposes only gets updated once a year.
When we back test our scoring engine and derived probability of defaults the results are less than desirable. There is at best a weak correlation between historical accounting information, the credit score and actual payment behavior. And it becomes self-evident when back tests are carried out on probability of default estimates. Original scoring models produced a binary output with 1 indicating client default and a zero indicating no default. They weren’t designed to calibrate with a graded distribution of defaults. This challenge was addressed by transforming the binary indicator into a probability of default estimates by applying a simple mathematical transformation to a revised credit score.
A scoring application centered on financial data will then only be able to run numbers once a year on audited financial statements. While customers are required by their terms sheets to share quarterly financial statements, credit departments generally don’t have the bandwidth to run analysis using these figures for the entire portfolio. The focus for that analysis are customers on the watch list, transactions in difficulty and classified relationships that are in the process of being worked out.
Compare this with the usage of Days Pass Due (DPD) data. DPD data is already available electronically as part of the accounting system. The collections group have in place processes that extract this data every month for using it as part of their customer follow up exercise. It gets updated as frequently as the payment frequency on the loan. And unlike financial data it has a clear linkage with a client’s ability and willingness to pay on time.
A DPD driven scoring engine provides a direct link to payment behavior. More importantly we instantly see a pick up in correlation between our data set, our scores and predicted defaults.
How does it work?
The objective of a scoring engine is to sift clients based on their predicted ability and willingness to service loans and repay principal amounts. This means whatever elements we use should be able to grade clients accordingly. For instance if we have two thousand corporate customers and ten credit grades then the distribution of customers across credit grades must be distributed according to some scheme. The scoring engine would be classified as a failure if it lumps all two thousand customers in one credit grade or even worse distributes them evenly across all grade without considering credit worthiness of future payment behavior.
Default experience is directly linked to realized payment behavior. Compared to other attributes and financial ratios, the correlation between payment behavior data and estimated probability of default is significantly higher.
A scoring engine then must meet certain criteria:
- It should not lump or clusters customers at common scoring thresholds. This happens when a large number of customers are assigned the same score because the scoring algorithm is not refined or selective. For example if the scoring engine uses three classes for quality of auditors and customer dataset includes only two of the three classes. In this instance the quality of auditor attribute will only allow us to break down our group in two categories, not ten grades. The same principle will hold true for industrial sectors and sub sectors, class of customers (SME, Corporate and Commercial). While great indicators for segmenting customers according to business and product lines, the ability of such indicators to predict customer default or repayment behavior is limited.
- The algorithm must segregate customers based on ability to pay. AAA rated customers should not exhibit behavior attributable to CCC- customers or vice versa. Which means that there must be some connection or feedback loop between rating, scoring variables and experienced behavior.
- Quite often scoring engines are tweaked to give preferential treatments to certain types or profiles of customers. This means that in addition to the ability to predict default, the engine is also acting as a filter for new business. Ideally this should be done outside the scope of the engine. We are not saying that preferred attribute ranges should not be given higher scores; for instance if you want to push for customers with coverage ratios above a certain threshold. However assigning SME customers or customers from a specific sector or segment of the economy because of a central bank or board directive should be avoided. The engine should be transparent and flexible enough to give higher exposure to elements because of their ability to predict default, not because of our desire to do business with holders of such attributes.
If the above design principles are followed when the distribution of customers across credit grades is displayed it will be skewed in the direction of preference encoded in the scoring engine. Skewed distributions are preferred over normalized distribution.
A DPD drive credit scoring model meets all three criteria. It leads to clustering only if customer payment behavior is clustered around certain dates (month ends, 60 or 90 days). It provides a direct link to realized payment history and most importantly it is transparent and flexible enough to be gauged and audited by outsiders.