My first interaction with a Monte Carlo simulation was not a very pleasant experience. It was an exam problem based on a difficult textbook and an even more incomprehensible study note that I had hardly understood. But over the years great teachers like Mark Broadie, friends like Carlos Desmaras and students at SP Jain and Alchemy platforms finally helped me crack the code.
This note starts with the basic concepts and ends with some fairly complex applications of Monte Carlo Simulations tools. The interest rate modeling piece is covered with a downloadable pdf file that Mark shared with us at Columbia as part of his course on Security pricing. Irrespective of how much I grow and write and teach it will be difficult for me to beat the simple elegance of his 10 pages on interest rate modelling.
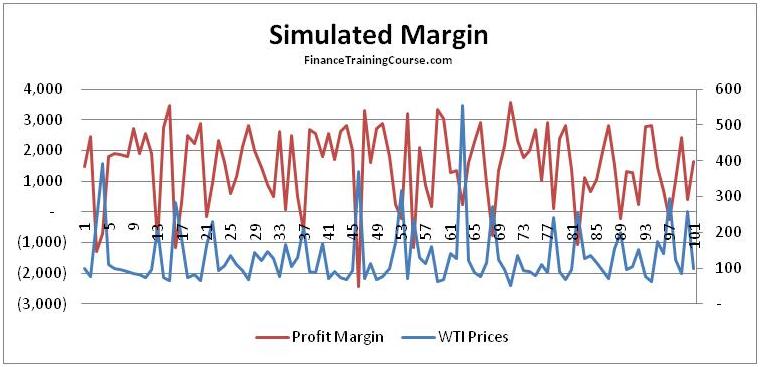
The key to building painless simulation models is spending time on understanding the process you are trying to model. Take a look at building financial models and linking the financial model posts below before you start to read up on Monte Carlo Simulations. The more time spent on design and structure, the more efficient your model and the quicker you can put it to work.
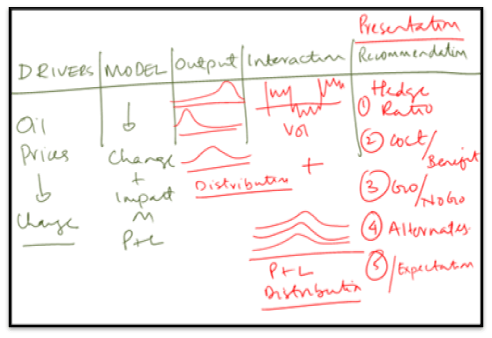
If as part of your initial analysis you have already identified the correct processes and model drivers, building the sheet becomes a great deal simpler.
Monte Carlo simulation. First steps and lessons.
- Monte-Carlo Simulation Excel– Building Equities, Commodities, Currencies and Interest Rate MC Simulators
- Building your first Monte Carlo (MC) simulator model for simulated equity prices in Excel
- Monte Carlo (MC) Simulation method: Understanding drift, diffusion and volatility drag
- Linking Monte Carlo Simulation, Binomial Trees and Black Scholes Equation
- Linking a financial model to your Monte Carlo Simulation
- Building Financial Models
While the first section focused on building model, the next section takes a step back and reviews the Black Scholes process. Taleb calls this understanding the generator function and the objective here to understand price behavior, more specifically price behavior for financial securities. We take a roundabout way of accomplishing this task by using the Black Scholes probabilities as our learning tool.
Understanding the Generator function.
The most complex application of Monte Carlo Simulation remains the modeling of interest rate behavior. We look at two different families of models. Cox Ingersoll Ross and Heath Jarrow & Merton.
Simulating Interest Rates using Monte Carlo Simulation Excel
- Simulating Interest Rates using trees and Monte Carlo Simulation
- Calibrating the Cox, Ingersoll, Ross (CIR) Interest Rate Simulator
- US Treasury Curve Data – Principal Component Analysis (PCA) process, data and volatility function
- Interest Rate Modeling: PCA analysis for HJM Interest Rate Simulation and calculating Eigenvectors
Comments are closed.